CoT3DRef: Chain-of-Thoughts Data-Efficient 3D Visual Grounding
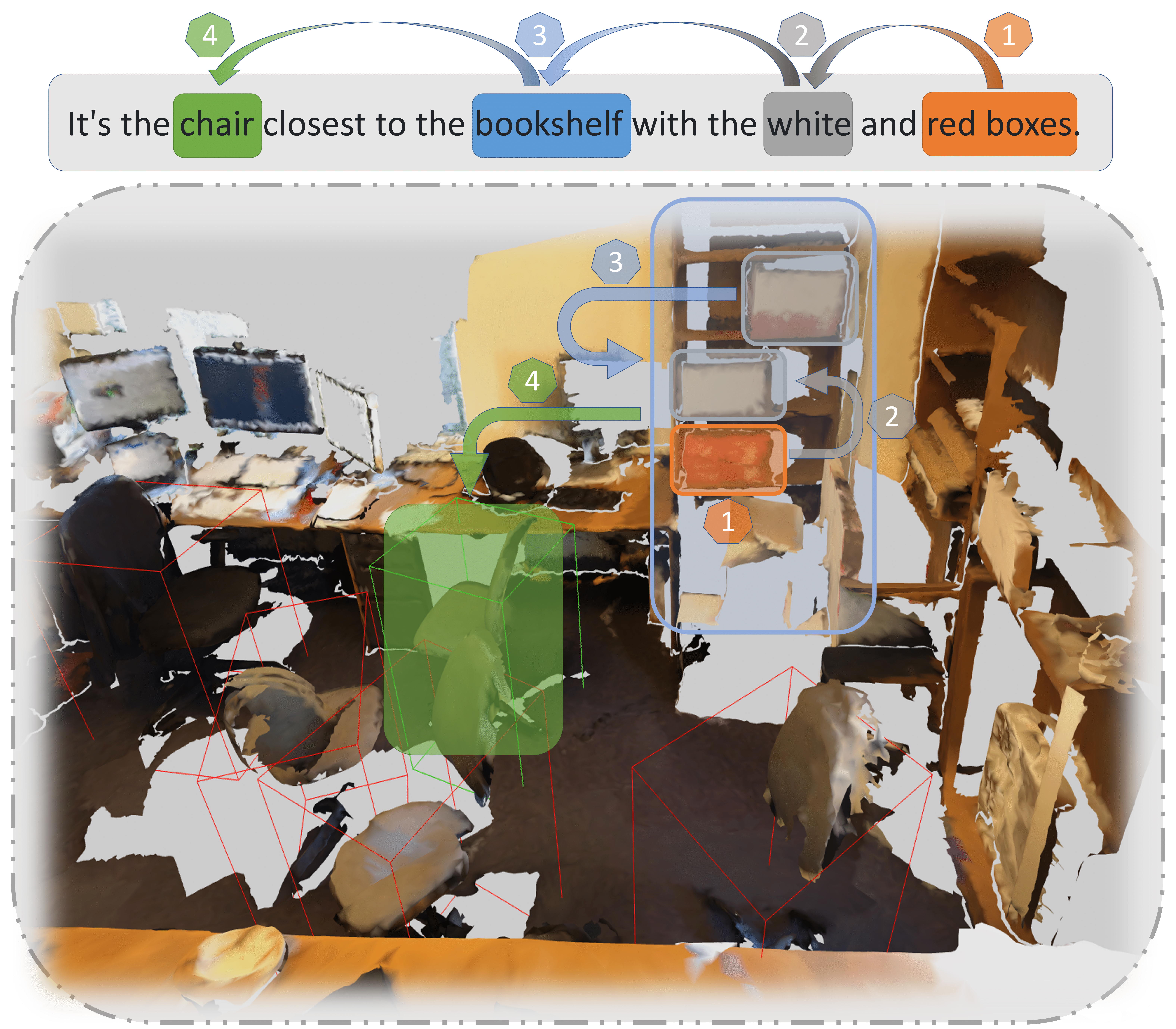
We aim to address the question of whether an interpretable 3D visual grounding framework, capable of emulating the human perception system, can be designed as shown in the figure above.
How Does CoT3DRef work?
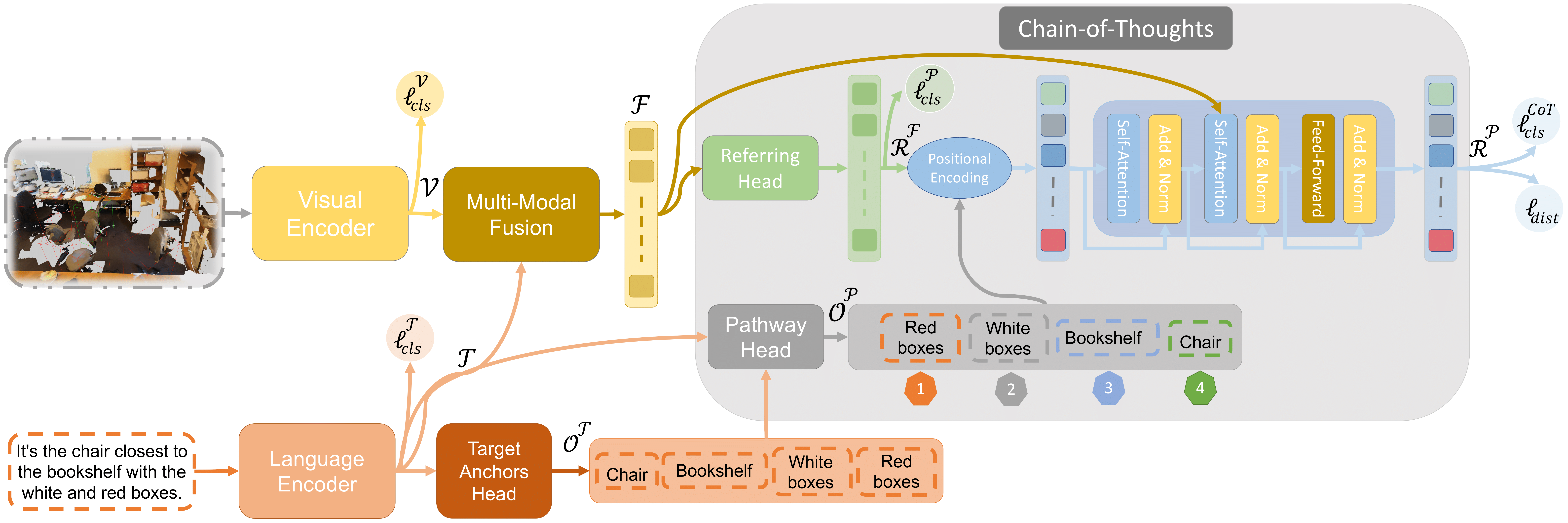
To achieve this objective, we formulate the 3D visual grounding problem as a sequence-to-sequence (Seq2Seq) task. As illustrated in the architecture above, the input sequence comprises 3D objects from the scene and an utterance describing a specific object. In contrast to existing architectures, our model predicts both the target object and a chain of anchors on the output side.
Data Data-Efficiency
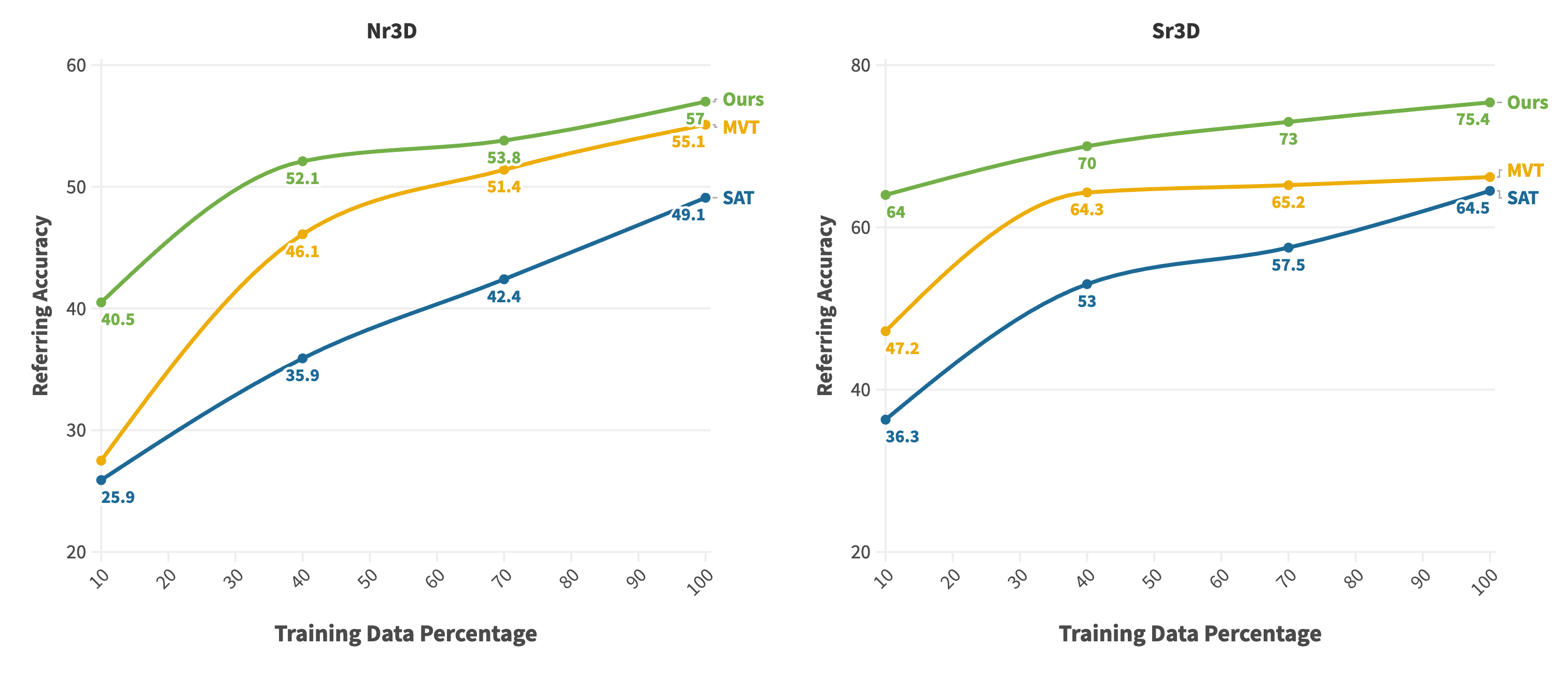
We evaluate our framework's effectiveness in a challenging scenario with limited training data. We test our model on four different percentages of data: 10%, 40%, 70%, and 100%. The figure above demonstrates that on the Sr3D dataset, even with only 10% of the data, our model achieves performance comparable to MVT and SAT models trained on 100% of the data. This result highlights the remarkable data efficiency of our model.